Genderless
Our group has produced several models and diagnostic methods for addressing gender bias in natural language processing and computer vision. Here we leverage our ICCV 2019 paper: Balanced Datasets Are Not Enough: Estimating and Mitigating Gender Bias in Deep Image Representations. In this paper we proposed a method to adversarially remove as much as possible from an image any features that could be predictive of whether a person will use a gendered word to describe it. We used a large dataset of images with captions and selected images that had references in the text such as "man" or "woman" and trained a model that can recognize the objects in the image but has as much difficulty as possible in predicting gender. When we applied this transformations to the image space, we can examine what the model is trying to do. Try your own images below and see what it does.
Here we leverage our ICCV 2019 paper: Balanced Datasets Are Not Enough: Estimating and Mitigating Gender Bias in Deep Image Representations to remove gender information -- as evidenced by textual references to gender-- using an adversarially trained neural network.

Original Image
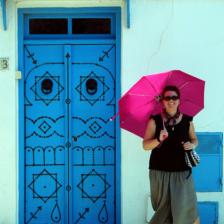
Genderless Image
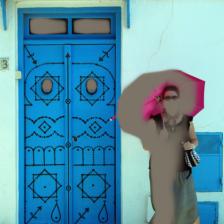